Why digital transformations fails:
Ignoring fundamental data quality issues
Many executives nod in agreement when discussing the value of data. “Data is incredibly valuable to our company,” they often say. “We’re part of a data-driven digital transformation.” According to this mindset, good data is good, and bad data is not so good. However, when it comes to creating an ontology—a sustainable solution to fundamental data quality issues—and they see the price the organization must pay, projects aimed at addressing these problems are often deprioritized.
The implicit message to the organization becomes: “It’s not that important.” As a result, by failing to tackle data problems at a fundamental level, companies will spend millions, even tens or hundreds of millions of euros, on digital transformations that are destined to fail in the long run.
"High-Quality Data is the foundation
of every successful AI-Powered Enterprise"
High-quality, accessible, and usable data is essential for an AI-powered enterprise. While machine learning can identify patterns in unstructured data, it cannot extract meaningful insights from low-quality or missing data. Data requires context; you can’t simply “point AI at your data,” as some in the field might claim. Depending on the use case, it may also be necessary to structure the data to make it compatible with different AI tools, including cognitive systems. More structure ensures that algorithms function more accurately and efficiently.
The hidden cost of poor data quality
For cognitive applications like chatbots, the training data required is the same information humans need—just in a different format and structure. To create predictive models, AI requires training data to build recognition patterns and examples to learn from. Data is more critical than the algorithms themselves; garbage in will always result in garbage out, even in a data-driven digital transformation.
Perhaps technology vendors have assured you that their AI solution seamlessly fits your data and business context. That’s optimistic. In reality, many AI solutions on the market are little more than attempts to patch gaps in data quality and missing context. Due to a lack of resources and poor data hygiene, organizations pay a high price—this includes the cost of using AI to fix flawed data, even though the real issues lie in the organization’s data processes and governance.
Yes, AI can help, but there’s more to it than what consultants and system integrators may have you believe.
The Ontology
A Rosetta Stone for Your Data
The solution to this problem is to harmonize your data with consistent data structures and models—creating a Rosetta Stone that helps your systems communicate and provides a beacon for AI to navigate your messy, fast-moving, diverse, unstructured, and structured data universe.
That Rosetta Stone is the ontology.
Note: The Rosetta Stone is a granodiorite stele inscribed with three scripts: Egyptian hieroglyphs, Demotic script, and Ancient Greek. Discovered in 1799 near the town of Rosetta (modern-day Rashid) during Napoleon’s campaign in Egypt, it was key to deciphering Egyptian hieroglyphs.
Author
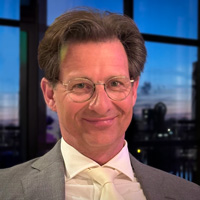
Erik Witte
CEO & Co-Founder
Erik is a seasoned executive and serial entrepreneur. Visionary thinking, connecting people to the vision and to each other is the essence of his professional career.